176 lines
7.8 KiB
Markdown
176 lines
7.8 KiB
Markdown
# Analysis of some predictions about the 2018 EA Survey
|
||
|
||
## Introduction.
|
||
Some effective altruists made predictions about the 2018 EA Survey: a survey which aims to reach most people within the effective altruism movement. Here, I present the set up for the prediction making, the questions, and explain some judgement calls I made when judging the answers. Everything is written such that you can play along. At the end, I provide some code to replicate my analysis.
|
||
|
||
## Set up
|
||
For every question, try to come up with an interval such that you're 80% confident the answer lies in it. If you use a search engine, the surveys from previous years are fair game.
|
||
|
||
## Judgement call
|
||
In some cases, people didn't answer the question. For example, under the is.veg variable, you can have TRUE, FALSE, or NA: Not Available. If their number is respectively x, y and z, it might be a good first order approximation to estimate the actual proportion of vegetarians/vegans as x/(x+y).
|
||
|
||
However, I've decided to be extremely anal about it, and choose to define the actual proportion of people who define as vegan as x/(x+y+z). I think that to do otherwise would be to replace questions. This doesn't make much of a difference in the case of plant eating, but it does in the identity politics questions. Curiously, doing so *raises* the average number of questions participants got right, but not by much.
|
||
|
||
Finally, I was told that the total number of people who answered the survey as 2607, so in the previous example, x+y+z := 2607. I stuck by 2607, yet the database I work with only has 2601 datapoints. I don't think this makes much of a difference either way.
|
||
|
||
## Questions
|
||
|
||
1. How many people do you think will take the EA survey?
|
||
|
||
1. What percent of people will say they got involved in EA in 2017?
|
||
|
||
1. What percent of people will say they first heard about EA from a personal contact?
|
||
|
||
1. What percent of people will say they first heard about EA from LessWrong?
|
||
|
||
1. What percent of people will say they are involved in a local EA group?
|
||
|
||
1. What percent of people will say they have taken the Giving What We Can pledge?
|
||
|
||
1. What percent of people will say they find the EA community either welcoming or very welcoming?
|
||
|
||
1. What percent of people will say they find the EA community either unwelcoming or very unwelcoming?
|
||
|
||
1. What percent of people will say that global poverty should be the top or near top priority?
|
||
|
||
1. What percent of people will say that cause prioritisation should be the top or near top priority?
|
||
|
||
1. What percent of people will say that reducing risks from AI should be the top or near top priority?
|
||
|
||
1. What percent of people will say that animal welfare/rights should be the top or near top priority?
|
||
|
||
1. What percent of people will say that meta charities should be the top or near top priority?
|
||
|
||
1. What percent of people who took the survey will be between 20 and 35 years of age?
|
||
|
||
1. What percent of people will identify as male?
|
||
|
||
1. What percent of people will identify as white?
|
||
|
||
1. What percent of people will say they live in the US or UK?
|
||
|
||
1. What percent of people will say they live in continental Europe?
|
||
|
||
1. What percent of people will say they are atheist, agnostic or non-religious?
|
||
|
||
1. What percent of people will say they are vegan or vegetarian?
|
||
|
||
1. What percent of people will say they are politically on the left or centre left?
|
||
|
||
1. What percent of people will say they are politically on the centre?
|
||
|
||
1. What percent of people will say they are politically on the right or centre right?
|
||
|
||
1. What percent of people will say that they are single?
|
||
|
||
1. What percent of people will say that they are employed?
|
||
|
||
1. What percent of people will say that they are a student?
|
||
|
||
## Answers
|
||
I got this answers using R from the data released by the EA survey people, available at [this link](https://github.com/peterhurford/ea-data/blob/master/data/2018/2018-ea-survey-anon-currencied-processed.csv). This allowed me to get a high level of precision, which was useful, because then I didn't have to care about whether the intervals were open or closed. For example, if someone gives an interval of (60,90), and the answer is 60, do I count it as right? If instead of 60 I have 59.76, I don't have to think about that problem.
|
||
|
||
1. 22.20943613
|
||
1. 33.17990027
|
||
1. 11.73762946
|
||
1. 15.53509781
|
||
1. 25.77675489
|
||
1. 59.37859609
|
||
1. 4.75642501
|
||
1. 61.33486766
|
||
1. 41.46528577
|
||
1. 44.1503644
|
||
1. 35.28960491
|
||
1. 34.06214039
|
||
1. NA
|
||
1. 59.76217875
|
||
1. 78.17414653
|
||
1. 52.35903337
|
||
1. NA
|
||
1. 72.0751822
|
||
1. 38.43498274
|
||
1. 60.98964327
|
||
1. 8.630609896
|
||
1. 2.685078634
|
||
1. 35.82662064
|
||
1. 52.5508247
|
||
1. 26.50556195
|
||
|
||
## Results
|
||
For the 35 people who took part in the original prediction making, their results can be seen in the following graphics:
|
||
|
||
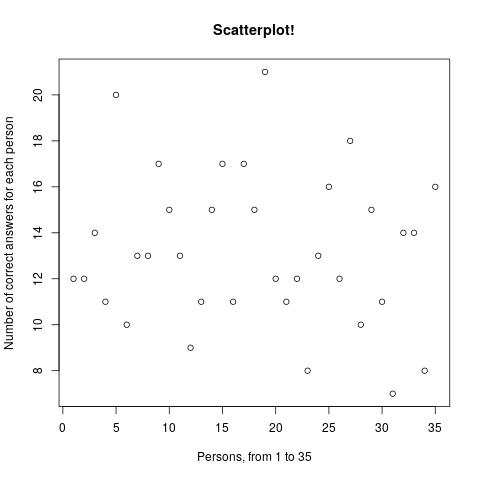
|
||
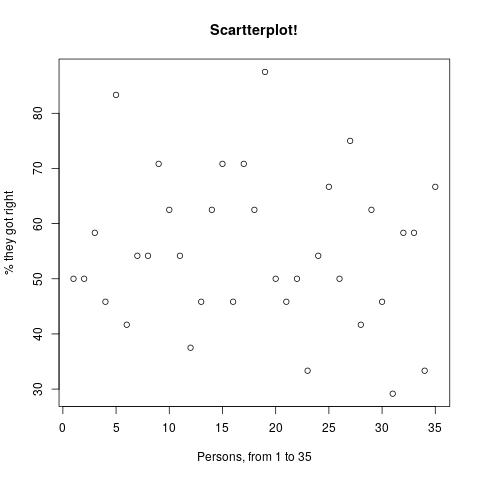
|
||
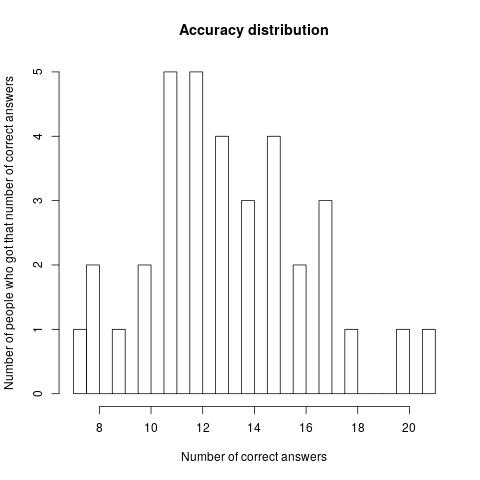
|
||
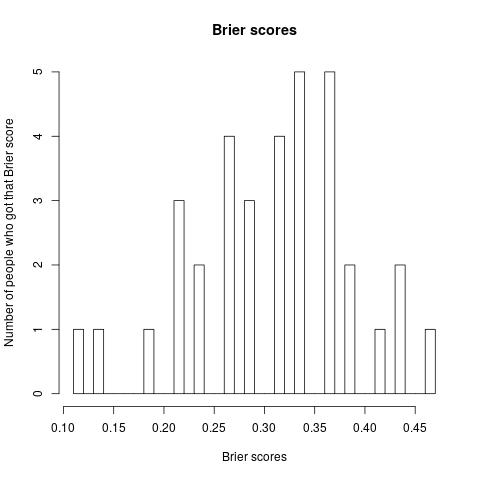
|
||
|
||
The average accuracy is 55.12%, that is, the average participant got 13.22 out of 24 questions right. If it had been reached, a target credence of 80% would imply an average of 19.2 correct answers. In other words, in this limited domain, when these people say 80%, the thing happens 55% of the time. If they bet, they'd be replacing ~1:1 bets with 1:4 bets.
|
||
|
||
## Further analysis
|
||
|
||
Questions for further analysis:
|
||
1. Should the two savants who got very near 80% be proud, or should we expect them to exist merely by chance?
|
||
2. Are the results an artifact of a small number of questions which were really hard (f.ex. the % of LessWrongers in EA)?
|
||
|
||
I expect to answer those questions in the near future.
|
||
|
||
|
||
## Code.
|
||
|
||
```
|
||
> ### We first read the data
|
||
> DataFrame <- read.csv(file="Predictions.csv", header=TRUE, sep=",")
|
||
> View(D)
|
||
>
|
||
> ### We then create a different object for storing the cleaned up data
|
||
> DataFrameProcessed=data.frame(matrix(nrow=35,ncol=52))
|
||
> LowerBoundsPersoni=NULL
|
||
>
|
||
> ### And clean up the data.
|
||
> for(i in c(1:35)){
|
||
+ as.numeric(strsplit(as.character(DataFrame[i,5]),", ")[[1]]) -> LowerBoundsPersoni
|
||
+ as.numeric(strsplit(as.character(DataFrame[i,6]),", ")[[1]]) -> UpperBoundsPersoni
|
||
>
|
||
+ for(j in c(1:26)){
|
||
+ DataFrameProcessed[i,(j*2)-1] <- LowerBoundsPersoni[j]
|
||
+ DataFrameProcessed[i,(j*2)] <- UpperBoundsPersoni[j]
|
||
+ }
|
||
+ }
|
||
> ### It shows that I've been programming in C.
|
||
>
|
||
> c(paste("Person-",c(1:35),sep=""))->rownames(DataFrameProcessed)
|
||
> c(rbind(paste("Q",c(1:26),"-lower",sep=""),paste("Q",c(1:26),"-upper",sep="")))->colnames(DataFrameProcessed)
|
||
> View(DataFrameProcessed)
|
||
>
|
||
> answers <- read.csv(file="answers.csv", header=TRUE, sep=",")[,2]
|
||
>
|
||
> ### Although every person answered every question, 2 anwers are not available.
|
||
> replaceNA <-function(x,y){
|
||
+ return( ifelse(is.na(x), y, x) )
|
||
+ }
|
||
>
|
||
> sum2<-function(x){ return(sum(replaceNA(x))) }
|
||
>
|
||
> ### Because some of the answers are not available, the comparison will give a NA. So we need sum2.
|
||
> total <- function(x){
|
||
+ y=c(1:26)*2
|
||
+ return(sum2(as.vector((answers>=DataFrameProcessed[x,y-1])) & as.vector(answers<=DataFrameProcessed[x,y])) )
|
||
+ }
|
||
>
|
||
> ### vapply applies a function to every member of a vector.
|
||
> vapply(c(1:35),total,numeric(1))->DataFrameProcessed$totalcorrect
|
||
>
|
||
> vapply(DataFrameProcessed$totalcorrect,Brierscore,numeric(1))->DataFrameProcessed$Brierscores
|
||
>
|
||
> ### And you can get graphics using
|
||
> png("Scatterplot3.png", units="px", width=3200, height=3200, res=500)
|
||
> plot(DataFrameProcessed$totalcorrect*100/24, xlab= "Persons, from 1 to 35", ylab="% of questions they got right", main="Scatterplot!")
|
||
> abline(h=mean(DataFrameProcessed$totalcorrect)*100/24, col="red")
|
||
> abline(h=80, col="blue")
|
||
> text(x=20, y=56, col="red", "Actual average")
|
||
> text(x=20, y=81, col="blue", "Target average")
|
||
> dev.off()
|
||
|
||
> ### As well as with the function hist(), whose parameter break = number allows you to control the granularity of the histogram.
|
||
´´´
|