Update readme.md
This commit is contained in:
parent
f4393e5bf4
commit
c98c90ea75
|
@ -1,3 +1,4 @@
|
|||
|
||||
# Data Analysis
|
||||
|
||||
## Index:
|
||||
|
@ -12,7 +13,20 @@
|
|||
3. Is more involvement with EA correlated with mental ilness?
|
||||
4. Is being mentally ill predictive of answering yes to: "Do you think you could personally benefit from EA community mental health resources?"
|
||||
5. Is having a mental disorder, or thinking you could potentially benefit from mental health ressources correlated with thinking that providing them is likely to be one of the most effective interventions available?
|
||||
6. Striving for Consistency. When is effective use of the EA Community's resources != the most effective interventions?
|
||||
6. When is an effective use of the EA Community's resources != the most effective intervention?
|
||||
7. How does further involment in EA correlate with opinions on the value of mental health resources?
|
||||
8. How does productivity lost because of mental health problems correlate with opinions on the value of mental health resources (and viceversa)?
|
||||
9. How does mental health affect productivity, and how is this mediated by access to healthcare?
|
||||
- 9.1. How does mental health affect productivity?
|
||||
- 9.2. How is lost productivity mediated by treatment and access to treatment?
|
||||
10. How does access to mental health ressources vary with a variety of factors?
|
||||
- 10.1. By countries or continent.
|
||||
- 10.2. By race/ethnicity.
|
||||
- 10.3. By mental health
|
||||
- 10.4. By age
|
||||
- 10.5. By involvement in EA.
|
||||
11. Insightful comments made by the respondents. [To be added]
|
||||
12. Conclusion, with some Fermi estimates. [To be added]
|
||||
|
||||
## 1. Is the population which answered the survey representative of EA overall?
|
||||
|
||||
|
@ -38,7 +52,7 @@ Initially, I was intending to find out the different mental disorder rates in th
|
|||
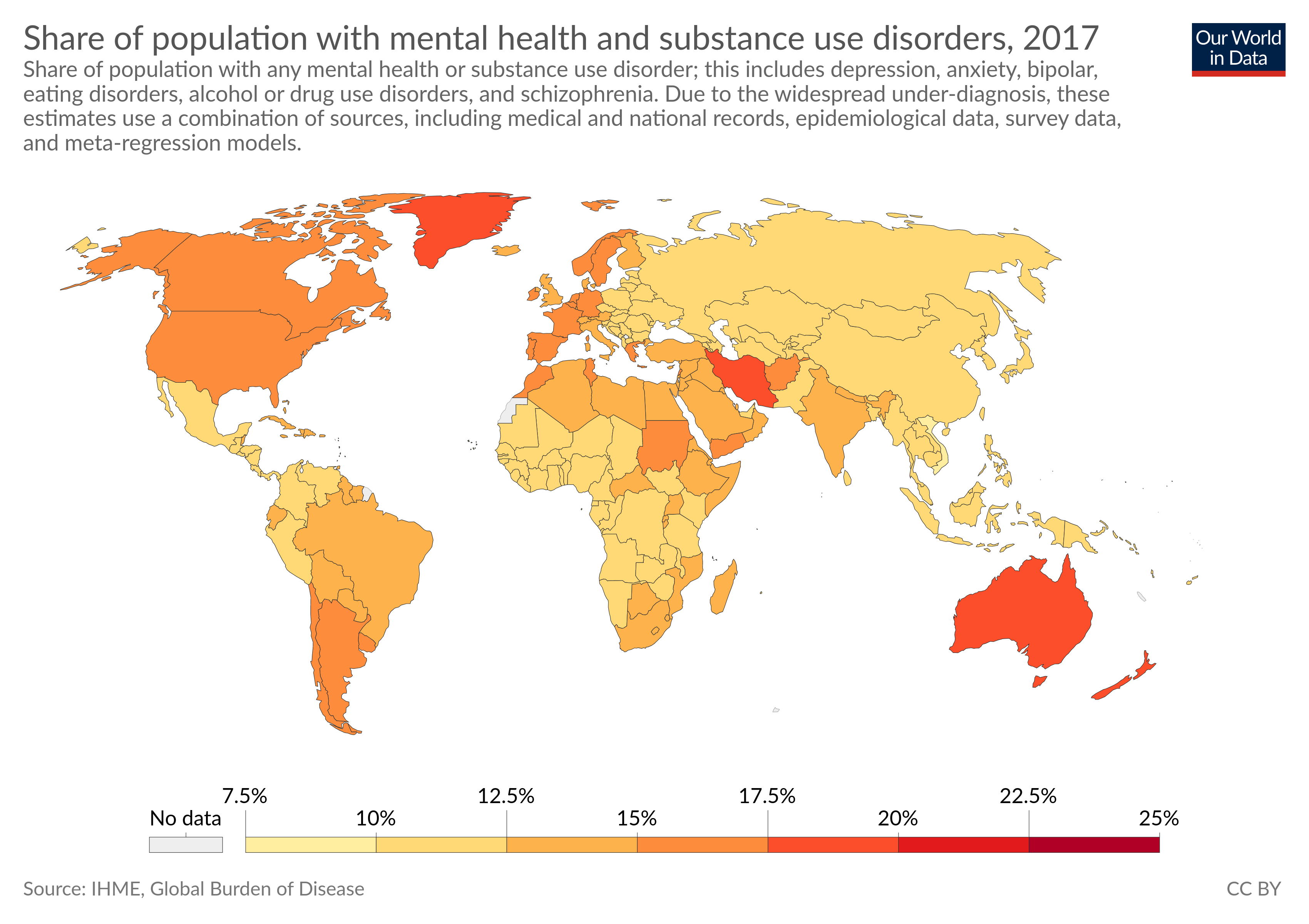
|
||||
|
||||
We see that the distribution is broadly similar across the countries among which EA has a presence. Most importantly, it doesn't surpass ~25% in any country, whereas among survey respondents:
|
||||
- 45% have been diagnosed with one or more mental disorders.
|
||||
- 45% have been diagnosed with one or more mental disorders (from our list).
|
||||
- 71% either have been diagnosed with one or more mental disorders, or intuit they have one.
|
||||
- The average number of mental ilnesses respondents have been diagnosed with is 0.82
|
||||
- The average number of mental ilnesses respondents have either been diagnosed with or intuit they have is 1.68. This number is higher than one because some (many respondents) have more than one disorder.
|
||||
|
@ -48,13 +62,13 @@ Thus, we can conclude with certainty that there are selection effects going on.
|
|||
## 3. Is more involvement with EA correlated with mental ilness?
|
||||
|
||||
The first four questions in our survey relate to involvement with EA:
|
||||
1. How involved are you in the Effective Altruism Community?
|
||||
2. Do you attend EA meetings?
|
||||
3. How much impact do EA ideas have on your life?
|
||||
4. Do you donate part of your income to GiveWell recommended charities?
|
||||
- How involved are you in the Effective Altruism Community?
|
||||
- Do you attend EA meetings?
|
||||
- How much impact do EA ideas have on your life?
|
||||
- Do you donate part of your income to GiveWell recommended charities?
|
||||
|
||||
And two measures of mental ilness:
|
||||
- A. A binary variable indicating whether a person was diagnosed with any mental ilness or not.
|
||||
- A. A binary variable indicating whether a person was diagnosed with any mental ilness (from our list) or not.
|
||||
- B. A binary variable indicating whether a person was diagnosed with any mental ilness / think they have a mental ilness, or not
|
||||
- C. An integer variable with the number of mental ilnesses a person has.
|
||||
- D. An integer variable with the number of mental ilnesses a person has or thinks they have.
|
||||
|
@ -68,13 +82,6 @@ For example, consider whether attending EA meeting has a positive effect on the
|
|||
```
|
||||
> summary(lm(A$m_ill_or_not ~ A$Do.you.attend.EA.meetings.))
|
||||
|
||||
Call:
|
||||
lm(formula = A$m_ill_or_not ~ A$Do.you.attend.EA.meetings.)
|
||||
|
||||
Residuals:
|
||||
Min 1Q Median 3Q Max
|
||||
-0.5238 -0.4375 -0.4359 0.5625 0.6667
|
||||
|
||||
Coefficients:
|
||||
Estimate Std. Error t value Pr(>|t|)
|
||||
(Intercept) 0.3333 0.2893 1.152 0.250
|
||||
|
@ -83,10 +90,6 @@ A$Do.you.attend.EA.meetings.No, but I regularly participate in an EA online grou
|
|||
A$Do.you.attend.EA.meetings.Yes, occasionally 0.1026 0.2948 0.348 0.728
|
||||
A$Do.you.attend.EA.meetings.Yes, often 0.1042 0.2926 0.356 0.722
|
||||
|
||||
Residual standard error: 0.501 on 298 degrees of freedom
|
||||
Multiple R-squared: 0.005741, Adjusted R-squared: -0.007604
|
||||
F-statistic: 0.4302 on 4 and 298 DF, p-value: 0.7868
|
||||
|
||||
```
|
||||
The key column is "Estimate". Smaller numbers are better, and we see that the more often one goes, the less likely is one to have been diagnosed with a mental ilness. No > No, but I regularly participate in an EA online group > Yes, occasionally > Yes often.
|
||||
|
||||
|
@ -96,9 +99,18 @@ The bottomline seems to be that EA is correlated with better mental health, acro
|
|||
|
||||
### 4. Is being mentally ill predictive of answering yes to: "Do you think you could personally benefit from EA community mental health resources?"
|
||||
|
||||
Yes, with p<0.001. In fact, p=6.93e-09. The obvious result is indeed obvious, and it serves as a proof of concept: we have enough power to find out *some* things.
|
||||
The two questions which directly ask about mental ilness are:
|
||||
- Which of these conditions have you been formally diagnosed with?
|
||||
- Which of these conditions do you think you may have, but have never been formally diagnosed with?
|
||||
|
||||
## 5. Is having a mental disorder, or thinking you could potentially benefit from mental health ressources correlated with thinking that providing them is likely to be one of the most effective interventions available?
|
||||
And the questions which ask about benefiting from mental health ressources are:
|
||||
- Do you think you could personally benefit from EA community mental health resources?
|
||||
- Which if any of the following resources do you think you could potentially benefit from?
|
||||
|
||||
I model having a mental ilness as before. Independent on the modelization, the answer our question is yes, people with mental ilnesses are more likely to think they can benefit from mental health ressources, with p-values ranging from 0.0004736 to
|
||||
7.612e-12. The obvious result is indeed obvious, and it serves as a proof of concept: we have enough power to find out *some* things.
|
||||
|
||||
## 5. Is having a mental disorder, or thinking you could potentially benefit from mental health ressources, correlated with thinking that providing them is likely to be one of the most effective interventions available?
|
||||
|
||||
Take a moment to make your predictions before you read ahead.
|
||||
|
||||
|
@ -111,24 +123,12 @@ Take a moment to make your predictions before you read ahead.
|
|||
|
||||
> summary(lm((A[,23]=="Agree" | A[,23]=="Strongly agree") ~ A[,26] == "Yes"))
|
||||
|
||||
Call:
|
||||
lm(formula = (A[, 23] == "Agree" | A[, 23] == "Strongly agree") ~
|
||||
A[, 26] == "Yes")
|
||||
|
||||
Residuals:
|
||||
Min 1Q Median 3Q Max
|
||||
-0.7188 -0.4286 0.2812 0.2812 0.5714
|
||||
|
||||
Coefficients:
|
||||
Estimate Std. Error t value Pr(>|t|)
|
||||
(Intercept) 0.42857 0.03612 11.864 < 2e-16 ***
|
||||
A[, 26] == "Yes"TRUE 0.29018 0.05558 5.221 3.32e-07 ***
|
||||
---
|
||||
Signif. codes: 0 ‘***’ 0.001 ‘**’ 0.01 ‘*’ 0.05 ‘.’ 0.1 ‘ ’ 1
|
||||
|
||||
Residual standard error: 0.4779 on 301 degrees of freedom
|
||||
Multiple R-squared: 0.08305, Adjusted R-squared: 0.08
|
||||
F-statistic: 27.26 on 1 and 301 DF, p-value: 3.321e-07
|
||||
```
|
||||
|
||||
I had forgotten how deep the waters are. If people think they can benefit from mental health ressources, the probability that they agree or strongly agree with the statement "I believe that offering mental health resources to its members is an effective use of the EA Community's resources" goes up from 42.857% to 29.018% + 42.857% = 71.875%, with a p-value of 3.321e-07!
|
||||
|
@ -138,14 +138,6 @@ Similarly, the probability that they agree with the statement "I believe that of
|
|||
```
|
||||
> summary(lm((A[,24]=="Agree" | A[,24]=="Strongly agree") ~ A[,26] == "Yes"))
|
||||
|
||||
Call:
|
||||
lm(formula = (A[, 24] == "Agree" | A[, 24] == "Strongly agree") ~
|
||||
A[, 26] == "Yes")
|
||||
|
||||
Residuals:
|
||||
Min 1Q Median 3Q Max
|
||||
-0.3257 -0.3257 -0.1641 0.6743 0.8359
|
||||
|
||||
Coefficients:
|
||||
Estimate Std. Error t value Pr(>|t|)
|
||||
(Intercept) 0.32571 0.03260 9.991 < 2e-16 ***
|
||||
|
@ -153,9 +145,6 @@ A[, 26] == "Yes"TRUE -0.16165 0.05016 -3.223 0.00141 **
|
|||
---
|
||||
Signif. codes: 0 ‘***’ 0.001 ‘**’ 0.01 ‘*’ 0.05 ‘.’ 0.1 ‘ ’ 1
|
||||
|
||||
Residual standard error: 0.4313 on 301 degrees of freedom
|
||||
Multiple R-squared: 0.03335, Adjusted R-squared: 0.03014
|
||||
F-statistic: 10.39 on 1 and 301 DF, p-value: 0.001409
|
||||
```
|
||||
|
||||
Two narratives present themselves:
|
||||
|
@ -191,7 +180,7 @@ The following table is the cold, raw, hard data for the graphic.
|
|||
Much could be said about how the above is why we take care of cultivating rationality.
|
||||
|
||||
## 6. Striving for Consistency. When is effective use of the EA Community's resources != the most effective interventions?
|
||||
According to my understanding of "effective" and "effective altruism", the following two questions are equivalent:
|
||||
According to my understanding of "effective" and "effective altruism", the following two questions have equivalent meanings:
|
||||
- "I believe that offering mental health resources to its members is an effective use of the EA Community's resources."
|
||||
- "I believe that offering mental health resources to effective altruists is NOT likely to be one of the most effective interventions available."
|
||||
|
||||
|
@ -200,3 +189,391 @@ However, I insisted in adding the second question because the connotations are s
|
|||
> - Source: The Power of Survey Design.
|
||||
|
||||
As it turns out, only 30% of respondents gave the same answer to the two questions. This is not correlated with mental disorders, age, sex, gender, impact of EA ideas in one's life or involvement in the EA community. It is, however, weakly correlated with donating to GiveWell recommended charities.
|
||||
|
||||
## 7. How does further involment in EA correlate with opinions on the value of mental health resources?
|
||||
The correlation between involvement in EA and having a positive opinion of providing mental health ressources is positive, but small and not significant at all. I wouldn't read anything into this.
|
||||
|
||||
The above is true both if we create a dummy for each possible answer (which are: "Strongly disagree", "Disagree", "", "Neutral/Not sure", "Agree", "Strongly agree") and if we instead convert the answers into a number ("Strongly disagree" = -2, "Disagree" = -1, ""=0, "Neutral/Not sure"=0, "Agree"=1, "Strongly agree"=2) and run a regression on that. For that matter, the switch function in R proved usatisfactory for working on strings; I wrote one more suited to my purposes and sufficient for n=303.
|
||||
|
||||
```
|
||||
switch2 <- function(Elements, Compare_With, Output_if_found, Output_if_Else){
|
||||
Lista = vector()
|
||||
for(element in Elements){
|
||||
i=1
|
||||
found=FALSE
|
||||
for(comparator in Compare_With){
|
||||
if(element == comparator){
|
||||
found = TRUE
|
||||
Lista = c(Lista, Output_if_found[i])
|
||||
}
|
||||
i =i +1
|
||||
}
|
||||
if(found == FALSE){
|
||||
Lista = c(Lista,Output_if_Else)
|
||||
}
|
||||
}
|
||||
return(Lista)
|
||||
}
|
||||
|
||||
## This function can then be used as follows:
|
||||
> colnames(A)[c(23,24)]
|
||||
[1] "X.I.believe.that.offering.mental.health.resources.to.its.members.is.an.effective.use.of.the.EA.Community.s.resources.."
|
||||
[2] "X.I.believe.that.offering.mental.health.resources.to.effective.altruists.is.NOT.likely.to.be.one.of.the.most.effective.interventions.available..."
|
||||
|
||||
switch2(A[,23], c("Strongly disagree", "Disagree", "Agree","Strongly agree"), c(-2,-1,1,2),0) -> Numerical_23
|
||||
switch2(A[,23], c("Strongly disagree", "Disagree", "Agree","Strongly agree"), c(-2,-1,1,2),0) -> Numerical_24
|
||||
|
||||
```
|
||||
|
||||
## 8. How does productivity lost because of mental health problems correlate with opinions on the value of mental health resources (and viceversa)?
|
||||
|
||||
The two questions which ask about productivity lost because of mental health problems are:
|
||||
- During the past 14 days, how many hours did you miss from work because of mental health problems? (numerical answer)
|
||||
- During the past 14 days, how much did mental health problems affect your productivity while you were working? (numerical answer)
|
||||
|
||||
And the two questions which ask about effectiveness of providing mental health ressources are:
|
||||
- "I believe that offering mental health resources to its members is an effective use of the EA Community's resources."
|
||||
- "I believe that offering mental health resources to effective altruists is NOT likely to be one of the most effective interventions available."
|
||||
|
||||
Under a wide range of modelizations / operationalizations, there is ~0 correlation between the two sets of answers. The one exception is if we consider a dummy variable for "hours lost due to mental ilness > 0". In that case, respondents who did lose >0 hours are somewhat more likely to support offering mental health resources, but this does not reach significant levels.
|
||||
|
||||
This is surprising, because a positive opinion of offering mental health resources is strongly correlated with thinking you could benefit from them, thinking you could benefit from them is strongly correlated with having a mental condition, and having a mental condition is strongly correlated with losing hours because of mental health.
|
||||
|
||||
Of course the relation "(significantly) correlated to" is not transitive in theory, and coming up with a nifty example is not difficult. But it is most curious that the relation is not transitive *in practice*.
|
||||
|
||||
```
|
||||
> colnames(A)[c(23,24,26)]
|
||||
[1] "X.I.believe.that.offering.mental.health.resources.to.its.members.is.an.effective.use.of.the.EA.Community.s.resources.."
|
||||
[2] "X.I.believe.that.offering.mental.health.resources.to.effective.altruists.is.NOT.likely.to.be.one.of.the.most.effective.interventions.available..."
|
||||
[3] "Do.you.think.you.could.personally.benefit.from.EA.community.mental.health.resources."
|
||||
|
||||
> summary(lm(Numerical_23 ~ A[,26]=="No"))
|
||||
## Significantly correlated, with p-value = 6.068e-07
|
||||
> summary(lm(A[,26] == "No" ~ A$m_ill_or_not))
|
||||
## Significantly correlated, with p-value = 0.0004736
|
||||
> summary(lm(A$m_ill_or_not ~ hours_lost))
|
||||
## Significantly correlated, with p-value = 5.479e-07
|
||||
> summary(lm(Numerical_23 ~ hours_lost))
|
||||
## Not significantly correlated!
|
||||
```
|
||||
|
||||
## 9. How does mental health affect productivity, and how is this mediated by access to healthcare?
|
||||
What is the effect of mental health on productivity, and how does access to healthcare mediate it?
|
||||
|
||||
### 9.1. How does mental health affect productivity?
|
||||
We can correlate our four markers of mental ilness on our two measures of lost productivity lost. However, the correlation runs both ways: from the data we cannot deduce whether people with mental conditions lose productivity, or whether losing productivity (i.e., losing a job) makes people more likely to be mentally ill.
|
||||
|
||||
If we assume that the effect is purely unidirectional (mental ilness -> lost productivity), three highlights are:
|
||||
- Being diagnosed with one or more mental conditions would cause a loss of ~9 hours per 2 weeks.
|
||||
- Each additional diagnosed mental ilness would cause a loss of ~5 hours per 2 weeks.
|
||||
- On a subjective 10 point scale, answers to the question ("During the past 14 days, how much did mental health problems affect your productivity while you were working?") increase by ~2 points if the respondent perceives they have at least 1 mental condition.
|
||||
|
||||
In total, we run 12 regressions, which are fully documented below. The casual reader is welcome to skip them, and continue to the next section.
|
||||
|
||||
```
|
||||
|
||||
# Call: lm(formula = A$m_ill_or_not ~ hours_lost)
|
||||
|
||||
Coefficients:
|
||||
Estimate Std. Error t value Pr(>|t|)
|
||||
(Intercept) 0.403430 0.030657 13.159 < 2e-16 ***
|
||||
hours_lost 0.009909 0.001886 5.254 2.94e-07 ***
|
||||
---
|
||||
Signif. codes: 0 ‘***’ 0.001 ‘**’ 0.01 ‘*’ 0.05 ‘.’ 0.1 ‘ ’ 1
|
||||
|
||||
|
||||
# Call: lm(formula = hours_lost ~ A$m_ill_or_not)
|
||||
|
||||
Coefficients:
|
||||
Estimate Std. Error t value Pr(>|t|)
|
||||
(Intercept) 2.010 1.168 1.721 0.0863 .
|
||||
A$m_ill_or_not 8.998 1.713 5.254 2.94e-07 ***
|
||||
---
|
||||
Signif. codes: 0 ‘***’ 0.001 ‘**’ 0.01 ‘*’ 0.05 ‘.’ 0.1 ‘ ’ 1
|
||||
|
||||
# Call: lm(formula = hours_lost ~ A$m_ill_or_not2)
|
||||
|
||||
Coefficients:
|
||||
Estimate Std. Error t value Pr(>|t|)
|
||||
(Intercept) 0.3951 1.6253 0.243 0.808
|
||||
A$m_ill_or_not2 8.1099 1.9224 4.219 3.32e-05 ***
|
||||
---
|
||||
Signif. codes: 0 ‘***’ 0.001 ‘**’ 0.01 ‘*’ 0.05 ‘.’ 0.1 ‘ ’ 1
|
||||
|
||||
# Call: lm(formula = hours_lost ~ A$m_ill_or_not + (A$m_ill_or_not2 &
|
||||
!A$m_ill_or_not))
|
||||
|
||||
Coefficients:
|
||||
Estimate Std. Error t value Pr(>|t|)
|
||||
(Intercept) 0.3951 1.5960 0.248 0.805
|
||||
A$m_ill_or_not 10.6125 2.0274 5.235 3.24e-07 ***
|
||||
A$m_ill_or_not2 & !A$m_ill_or_notTRUE 3.4571 2.3352 1.480 0.140
|
||||
---
|
||||
Signif. codes: 0 ‘***’ 0.001 ‘**’ 0.01 ‘*’ 0.05 ‘.’ 0.1 ‘ ’ 1
|
||||
|
||||
# Call: lm(formula = hours_lost ~ A$num_mental_ilnesses)
|
||||
|
||||
Coefficients:
|
||||
Estimate Std. Error t value Pr(>|t|)
|
||||
(Intercept) 2.174 1.051 2.069 0.0395 *
|
||||
A$num_mental_ilnesses 4.856 0.767 6.331 9.58e-10 ***
|
||||
---
|
||||
Signif. codes: 0 ‘***’ 0.001 ‘**’ 0.01 ‘*’ 0.05 ‘.’ 0.1 ‘ ’ 1
|
||||
|
||||
# Call: lm(formula = hours_lost ~ A$num_mental_ilnesses2)
|
||||
|
||||
Coefficients:
|
||||
Estimate Std. Error t value Pr(>|t|)
|
||||
(Intercept) -0.6053 1.2060 -0.502 0.616
|
||||
A$num_mental_ilnesses2 4.0640 0.5317 7.643 3.33e-13 ***
|
||||
---
|
||||
Signif. codes: 0 ‘***’ 0.001 ‘**’ 0.01 ‘*’ 0.05 ‘.’ 0.1 ‘ ’ 1
|
||||
|
||||
# Call: lm(formula = hours_lost ~ A$num_mental_ilnesses + A$num_mental_ilnesses2)
|
||||
|
||||
Coefficients:
|
||||
Estimate Std. Error t value Pr(>|t|)
|
||||
(Intercept) -0.5355 1.2055 -0.444 0.657
|
||||
A$num_mental_ilnesses 1.4484 1.0981 1.319 0.188
|
||||
A$num_mental_ilnesses2 3.3057 0.7826 4.224 3.25e-05 ***
|
||||
---
|
||||
Signif. codes: 0 ‘***’ 0.001 ‘**’ 0.01 ‘*’ 0.05 ‘.’ 0.1 ‘ ’ 1
|
||||
|
||||
# Call: lm(formula = A$m_ill_or_not ~ effect_while_working)
|
||||
|
||||
Coefficients:
|
||||
Estimate Std. Error t value Pr(>|t|)
|
||||
(Intercept) 0.10805 0.04885 2.212 0.0278 *
|
||||
effect_while_working 0.08744 0.01020 8.577 5.56e-16 ***
|
||||
---
|
||||
Signif. codes: 0 ‘***’ 0.001 ‘**’ 0.01 ‘*’ 0.05 ‘.’ 0.1 ‘ ’ 1
|
||||
|
||||
# Call: lm(formula = effect_while_working ~ A$m_ill_or_not)
|
||||
|
||||
Coefficients:
|
||||
Estimate Std. Error t value Pr(>|t|)
|
||||
(Intercept) 3.0062 0.1806 16.644 < 2e-16 ***
|
||||
A$m_ill_or_not 2.2764 0.2654 8.577 5.56e-16 ***
|
||||
---
|
||||
Signif. codes: 0 ‘***’ 0.001 ‘**’ 0.01 ‘*’ 0.05 ‘.’ 0.1 ‘ ’ 1
|
||||
|
||||
# Call: lm(formula = effect_while_working ~ A$m_ill_or_not2)
|
||||
|
||||
Coefficients:
|
||||
Estimate Std. Error t value Pr(>|t|)
|
||||
(Intercept) 1.9518 0.2402 8.126 1.22e-14 ***
|
||||
A$m_ill_or_not2 2.9226 0.2828 10.335 < 2e-16 ***
|
||||
---
|
||||
Signif. codes: 0 ‘***’ 0.001 ‘**’ 0.01 ‘*’ 0.05 ‘.’ 0.1 ‘ ’ 1
|
||||
|
||||
# Call: lm(formula = effect_while_working ~ A$num_mental_ilnesses)
|
||||
|
||||
Coefficients:
|
||||
Estimate Std. Error t value Pr(>|t|)
|
||||
(Intercept) 3.1341 0.1630 19.228 <2e-16 ***
|
||||
A$num_mental_ilnesses 1.1130 0.1185 9.394 <2e-16 ***
|
||||
---
|
||||
Signif. codes: 0 ‘***’ 0.001 ‘**’ 0.01 ‘*’ 0.05 ‘.’ 0.1 ‘ ’ 1
|
||||
|
||||
# Call: lm(formula = effect_while_working ~ A$num_mental_ilnesses2)
|
||||
|
||||
Coefficients:
|
||||
Estimate Std. Error t value Pr(>|t|)
|
||||
(Intercept) 2.43830 0.18171 13.42 <2e-16 ***
|
||||
A$num_mental_ilnesses2 0.95910 0.07998 11.99 <2e-16 ***
|
||||
---
|
||||
Signif. codes: 0 ‘***’ 0.001 ‘**’ 0.01 ‘*’ 0.05 ‘.’ 0.1 ‘ ’ 1
|
||||
```
|
||||
|
||||
### 9.2. How is this mediated by treatment and access to treatment?
|
||||
So unsurprisingly, having a mental ilness decreases both work hours, and self-reported productivity when working. Thus, a pressing question is whether therapy has an effect on that.
|
||||
|
||||
Four questions ask about treatment or access to treatment:
|
||||
- How challenging was it to receive the mental healthcare services you needed within the past 12 months?
|
||||
- How challenging is it to find useful information on mental healthcare services?
|
||||
- "I am currently receiving the mental healthcare I need."
|
||||
- How satisfied are you with the mental healthcare you've received?
|
||||
|
||||
And we can correlate their answers with our measures for lost productivity.
|
||||
- During the past 14 days, how many hours did you miss from work because of mental health problems? (numerical answer)
|
||||
- During the past 14 days, how much did mental health problems affect your productivity while you were working? (numerical answer)
|
||||
|
||||
#### 9.2.1. Mediated by answers to "I am currently receiving the mental healthcare I need".
|
||||
|
||||
Respondents could choose one of the following options, after being prompted by the sentence "I am currently receiving the mental healthcare I need":
|
||||
```
|
||||
[1] "Strongly agree"
|
||||
[2] "Agree"
|
||||
[3] "Strongly disagree"
|
||||
[4] "Disagree"
|
||||
[5] "I do not currently need mental healthcare."
|
||||
[6] ""
|
||||
```
|
||||
|
||||
We can thus define two dummy variables and regress on them.
|
||||
```
|
||||
> Receiving_positive = A[,19]%in%c("Agree", "Strongly agree")*1 ## 1/TRUE if the respondent thinks they're receiving the mental healthcare they need, 0/FALSE otherwise. Note that this excludes respondents who feel that they don't need mental healthcare.
|
||||
> Receiving_negative= A[,19]%in%c("Disagree", "Strongly disagree")*1 ## 1/TRUE if the respondent thinks they're not receiving the mental healthcare they need, 0/FALSE otherwise.
|
||||
```
|
||||
|
||||
The results seem to indicate that when respondents receive the mental health care they think they need, they improve. Note that we care about how much. Note as well that both of the coefficients are positive because the intercept refers to respondents who did not feel they needed mental healthcare.
|
||||
```
|
||||
> summary(lm(hours_lost ~ Receiving_positive + Receiving_negative))
|
||||
|
||||
Call:
|
||||
lm(formula = hours_lost ~ Receiving_positive + Receiving_negative)
|
||||
|
||||
Coefficients:
|
||||
Estimate Std. Error t value Pr(>|t|)
|
||||
(Intercept) 0.6435 1.3885 0.463 0.643388
|
||||
Receiving_positiveTRUE 7.7986 2.0297 3.842 0.000151 ***
|
||||
Receiving_negativeTRUE 10.3071 2.1209 4.860 1.96e-06 ***
|
||||
---
|
||||
Signif. codes: 0 ‘***’ 0.001 ‘**’ 0.01 ‘*’ 0.05 ‘.’ 0.1 ‘ ’ 1
|
||||
|
||||
> summary(lm(effect_while_working ~ Receiving_positive + Receiving_negative))
|
||||
|
||||
Call:
|
||||
lm(formula = effect_while_working ~ Receiving_positive + Receiving_negative)
|
||||
|
||||
Coefficients:
|
||||
Estimate Std. Error t value Pr(>|t|)
|
||||
(Intercept) 2.4818 0.2123 11.689 < 2e-16 ***
|
||||
Receiving_positiveTRUE 2.1182 0.3077 6.884 3.5e-11 ***
|
||||
Receiving_negativeTRUE 2.9386 0.3185 9.227 < 2e-16 ***
|
||||
---
|
||||
Signif. codes: 0 ‘***’ 0.001 ‘**’ 0.01 ‘*’ 0.05 ‘.’ 0.1 ‘ ’ 1
|
||||
|
||||
```
|
||||
|
||||
Notice that the regression can be rewritten as:
|
||||
|
||||
> hours_lost ~ Dummy_for(Thinks they need some kind of healthcare) + Dummy_for(Thinks they need some kind of healthcare)\*Dummy_for(Is receiving the mental healthcare they think they need).
|
||||
> And that Dummy_for(Thinks they need some kind of healthcare) ~ Dummy(Has a mental condition)
|
||||
|
||||
If this was fully rigorous, giving people "the mental healthcare they need" would reduce hours lost per two weeks from 10.3071 to 7.7986, that is, a difference of 2.5 hours per two weeks, or 1.25 hours per week. Results are similar if we:
|
||||
- ask instead "How satisfied are you with the mental healthcare you've received?"
|
||||
- exclude people who did not feel they needed mental healthcare (n=191).
|
||||
- and further restrict the regression to respondents thought they had a mental condition from our list, or who had been diagnosed with one (n=175).
|
||||
- or restrict it to people (who claim to have been) diagnosed with a mental condition (n=129).
|
||||
|
||||
In this last case, the implied effect doubles, to 2.2 hours saved per week, as seen below.
|
||||
|
||||
```
|
||||
> m_ill3= (Receiving_positive | Receiving_negative) & A$m_ill_or_not
|
||||
> sum(m_ill3)
|
||||
[1] 124
|
||||
> summary(lm(hours_lost[m_ill3] ~ Receiving_positive[m_ill3]))
|
||||
|
||||
Call:
|
||||
lm(formula = hours_lost[m_ill3] ~ Receiving_positive[m_ill3])
|
||||
|
||||
Coefficients:
|
||||
Estimate Std. Error t value Pr(>|t|)
|
||||
(Intercept) 14.833 3.086 4.807 4.67e-06 ***
|
||||
Receiving_positive[m_ill3]TRUE -4.428 4.018 -1.102 0.273
|
||||
---
|
||||
Signif. codes: 0 ‘***’ 0.001 ‘**’ 0.01 ‘*’ 0.05 ‘.’ 0.1 ‘ ’ 1
|
||||
```
|
||||
|
||||
Note that, in none of the above cases we've gotten a significant effect. This is because outliers, for example, respondents who lost a job because of mental ilness, and thus ~40 hours per week, make the error bars huge. In this case, excluding outliers doesn't make sense at all; instead, knowing where the error comes from, I think it makes sense to not get hung up on p-values. As a proof of concept, here is the same regression as above, but considering the logarithm of hours lost (which gives less weigh to outliers); we "suddenly" reach significance (p-value of 0.00698). On the flipside, the logarithm of hours lost is not an intuitive unit in which to report results.
|
||||
|
||||
```
|
||||
> summary(lm(log(hours_lost[m_ill3]+1) ~ Receiving_positive[m_ill3]))
|
||||
Coefficients:
|
||||
Estimate Std. Error t value Pr(>|t|)
|
||||
(Intercept) 2.0578 0.1994 10.322 < 2e-16 ***
|
||||
Receiving_positive[m_ill3]TRUE -0.7131 0.2596 -2.747 0.00698 **
|
||||
---
|
||||
Signif. codes: 0 ‘***’ 0.001 ‘**’ 0.01 ‘*’ 0.05 ‘.’ 0.1 ‘ ’ 1
|
||||
```
|
||||
|
||||
In the ideal case, if information was free, what I'd like to have is a randomized trial in which:
|
||||
- Productivity and severity of mental ilness are measured across a population
|
||||
- Mental healthcare is provided to a randomly selected part of the population; the treatment group.
|
||||
- Part of the treatment group takes up that freely provided mental heathcare, and part of the control group pays for the treatment out of pocket.
|
||||
- Some time passes.
|
||||
- Productivity and severity of mental ilness are measured again, both in the control and treatment group.
|
||||
|
||||
Alas, we don't have that, and thus cannot take the above crosssections at face value. Imagine, for example, that people with lighter mental conditions find it easier to deal with the hassle of finding good treatment, which seems plausible. In that case, the causal arrow would go both ways: (getting good mental healthcare -> healing occurs -> people lose less hours each week), but also (having a lighter mental ilness -> easier to find good mental healthcare) + (having a lighter mental ilness -> loose less work hours). It could also be that, as people regress to the mean with respect to their mental ilness, and become happier, they attribute their improvement to whatever therapy they were receiving, and become more satisfied with it. If we assume that the bias goes in the direction of amplifying the effect of good mental healthcare, the above figures become an upper estimate of its effect.
|
||||
|
||||
|
||||
## 10. How does access to mental health ressources vary with a wide variety of factors?
|
||||
|
||||
Acess is asked about in the following two questions:
|
||||
14. How challenging was it to receive the mental healthcare services you needed within the past 12 months?
|
||||
15. How challenging is it to find useful information on mental healthcare services?
|
||||
|
||||
The main limitation of these questions is that the answers will depend on the subjective experience and capabilities of the respondents.
|
||||
|
||||
### 10.1. By countries or continent.
|
||||
With 52 countries and 303 respondents, we don't have the power to say anything. We still don't have anything to say if we aggregate by continent instead, except that unsuprisingly, respondents find it more difficult to access mental healthcare in Africa than in any other continent. However, the effect was not significant, given that very few respondents were from Africa.
|
||||
|
||||
### 10.2. By race/ethnicity.
|
||||
Perhaps unsurprisingly, white people find it less challenging to receive mental healthcare, and to find information about it. The effect is magnified when considering only respondents with a mental condition.
|
||||
```
|
||||
> summary(lm(switch2(A[,16][M_ILL2], c("Very easy", "Fairly easy", "Moderate", "Fairly hard", "Very hard"), c(3,1,-1,-3),0) ~ A$Race.ethnicity[M_ILL2]))
|
||||
|
||||
Coefficients:
|
||||
Estimate Std. Error t value Pr(>|t|)
|
||||
(Intercept) -1.6667 1.1053 -1.508 0.134
|
||||
A$Race.ethnicity[M_ILL2]Asian 0.9167 1.2960 0.707 0.481
|
||||
A$Race.ethnicity[M_ILL2]Hispanic or Latino/a/x 0.6667 2.2105 0.302 0.763
|
||||
A$Race.ethnicity[M_ILL2]Mixed race/Multi-racial 1.1667 1.4621 0.798 0.426
|
||||
A$Race.ethnicity[M_ILL2]White 1.7576 1.1178 1.572 0.118
|
||||
|
||||
|
||||
> summary(lm(switch2(A[,16], c("Very easy", "Fairly easy", "Moderate", "Fairly hard", "Very hard"), c(3,1,-1,-3),0) ~ A$Race.ethnicity))
|
||||
|
||||
Coefficients:
|
||||
Estimate Std. Error t value Pr(>|t|)
|
||||
(Intercept) -0.41667 0.39899 -1.044 0.297
|
||||
A$Race.ethnicityAsian 0.15580 0.49218 0.317 0.752
|
||||
A$Race.ethnicityBlack 0.41667 1.05562 0.395 0.693
|
||||
A$Race.ethnicityHispanic or Latino/a/x 0.21667 0.73569 0.295 0.769
|
||||
A$Race.ethnicityMiddle Eastern 0.41667 1.05562 0.395 0.693
|
||||
A$Race.ethnicityMixed race/Multi-racial 0.08333 0.69106 0.121 0.904
|
||||
A$Race.ethnicityWhite 0.46730 0.40896 1.143 0.254
|
||||
```
|
||||
|
||||
### 10.3. By mental health
|
||||
Among all our markers, having worse mental health is correlated with finding it more challenging to receive mental healthcare, and to find information about it. Sometimes the effect is significant, p<0.05, but it it is never large (the largest is a change of -0.16 in a -3 to +3 scale)
|
||||
|
||||
### 10.4. By age
|
||||
No effect.
|
||||
|
||||
### 10.5. By involvement in EA.
|
||||
No effect.
|
||||
|
||||
|
||||
## Inf. Survey questions
|
||||
1. How involved are you in the Effective Altruism Community?
|
||||
2. Do you attend EA meetings?
|
||||
3. How much impact do EA ideas have on your life?
|
||||
4. Do you donate part of your income to GiveWell recommended charities?
|
||||
5. Which of these conditions have you been formally diagnosed with?
|
||||
6. Which of these conditions do you think you may have, but have never been formally diagnosed with?
|
||||
7. During the past 14 days, how many hours did you miss from work because of mental health problems?
|
||||
8. During the past 14 days, how many hours did you miss from work because of any other reason, such as vacation, other commitments, etc.?
|
||||
9. During the past 14 days, about how many hours did you actually work?
|
||||
10. During the past 14 days, how much did mental health problems affect your productivity while you were working?
|
||||
11. During the past 14 days, how much did mental health problems affect your ability to do your regular daily activities, other than work at a job?
|
||||
12. Are the past 14 days representative of your average mental health?
|
||||
13. If you answered "No" to the previous question, how has your mental health differed over the past 14 days?
|
||||
14. How challenging was it to receive the mental healthcare services you needed within the past 12 months?
|
||||
15. How challenging is it to find useful information on mental healthcare services?
|
||||
16. Do you experience financial difficulties as a result of mental healthcare?
|
||||
17. "I am currently receiving the mental healthcare I need."
|
||||
18. How satisfied are you with the mental healthcare you've received?
|
||||
19. Which of the following treatments have you tried?
|
||||
20. Any other thoughts you'd like to share on mental healthcare access?
|
||||
21. "I believe that offering mental health resources to its members is an effective use of the EA Community's resources."
|
||||
22. "I believe that offering mental health resources to effective altruists is NOT likely to be one of the most effective interventions available."
|
||||
23. Please explain your answers to the questions above.
|
||||
24. Do you think you could personally benefit from EA community mental health resources?
|
||||
25. Which (if any) of the following resources do you think you could potentially benefit from?
|
||||
26. Any other thoughts you'd like to share on EA-sponsored mental health resources?
|
||||
27. Which country do you live in?
|
||||
28. What is your age?
|
||||
29. What is your gender?
|
||||
30. What is your race/ethnicity?
|
||||
|
|
Loading…
Reference in New Issue
Block a user